- Analytics Wisdom
- Posts
- Cracking the Code of Customer Loyalty: Cohort Analysis Insights for E-commerce Success: Part 1
Cracking the Code of Customer Loyalty: Cohort Analysis Insights for E-commerce Success: Part 1
How to Use Cohort Analysis to Drive Long-Term Customer Loyalty and Revenue Growth in E-commerce

Why ? What’s the point?
In this article, we embark on a journey into the world of cohort analysis and explore how it holds the potential to revolutionize the landscape of e-commerce. For those unfamiliar with cohort analysis + a bonus with the SQL code for it, refer my previous article
In the dynamic world of e-commerce, where customers have seemingly endless options at their fingertips, achieving and sustaining customer loyalty has become a strategic imperative. For e-commerce businesses, understanding customer behavior and retention is not just a matter of curiosity but a mission-critical pursuit. This is where cohort analysis emerges as a game-changing tool that can transform the outcomes of e-commerce operations.
Cohort analysis, a sophisticated data analysis technique, has gained prominence as an invaluable resource for deciphering customer patterns, enhancing user retention, and ultimately, driving sustained growth. It offers a profound insight into how different groups of customers behave over time, enabling businesses to tailor their strategies for maximum impact.
Key Components of Cohort Analysis in E-commerce:
Cohort Formation: The first step in cohort analysis is defining the criteria for cohort creation. For example, an e-commerce business might create cohorts based on the month in which customers made their first purchase. Each cohort will represent customers who made their initial purchase during a specific month.
Tracking Metrics: Once cohorts are defined, businesses track key performance metrics over time for each cohort. Common metrics include customer retention rates, average order value, and revenue per user.
Time Periods: Cohort analysis involves analyzing cohorts over predefined time periods, typically months or quarters. This allows businesses to observe how customer behavior evolves over time within each cohort.
Comparative Analysis: The primary goal of cohort analysis is to compare the performance of different cohorts. By doing so, businesses can identify trends and patterns that can inform strategic decisions.
Analyzing a Case Study with Cohorts
In this case study, we're about to take a closer look at the data for a small e-commerce store. The shop sells wall art to techo enthusiasts. Our mission is to employ cohort analysis to decipher the store's customer behavior and uncover actionable insights that will aid its growth and success.
We’ll plot the analysis using shades of green, the more concentrated the higher the number and vice versa.
Retention Rate Cohort
In this specific context, we've performed a time-based cohort analysis for an e-commerce store, categorizing customers by the month of their first purchase.

What key takeaways do we deduce from this heatmap?
Initial Cohorts Have Lower Retention: The earliest cohorts (e.g., 2020-01) show relatively lower retention rates in the subsequent months. This could be due to various reasons, such as initial customers being early adopters who might not have stuck around, or perhaps the store made improvements over time, leading to better retention of later cohorts.
Increasing Retention Over Time: Cohorts from later months (e.g., 2020-09, 2020-10) show significantly higher retention rates compared to the earlier cohorts. This deduces that the store has made improvements in its offerings, customer service, or other aspects leading to higher customer loyalty.
Consistent Growth: The number of customers and their retention rates generally increase month over month, suggesting that the store is successfully attracting and retaining more customers over time.
Spotty Data for Recent Cohorts: The most recent cohorts (e.g., 2020-11, 2020-12) have fewer cohort indices, reflecting that these cohorts have not been around long enough to produce retention data for many subsequent months.
Notable Retention Spikes: Some cohorts show a spike in retention during certain months. For example, the 2020-08 cohort shows a spike in its 3rd month. Such spikes might be due to seasonal trends, promotions, or other events that have re-engaged customers.
Recommendations:
Engage Early Adopters: The earliest cohorts showed relatively lower retention rates in subsequent months. Consider reaching out to these early adopters with special offers or surveys to understand their initial experiences and reasons for not returning. They can provide valuable feedback to improve the shopping experience.
Leverage Seasonal Trends: Some cohorts showed a spike in retention during certain months. Identify these seasonal trends and capitalize on them by offering promotions, seasonal products, or themed marketing campaigns.
Customer Journey Cohort Analysis
In this kind of an analysis, customers are usually grouped based on their initial interaction with the store. This could be the month they first visited, clicked on a product, or added an item to their cart.
Here’s how those cohorts behave over time for our store.

Damn thats a lot of graphs
What key takeaways do we deduce from this heatmap?
Initial Drop-off is Significant: The first stage, "Landing", naturally has a 100% retention rate as it represents customers landing on the website. However, as they progress through the stages, there is a significant drop-off. This is common in e-commerce, where many visitors browse but don't necessarily intend to make a purchase.
High Retention from "Add to Cart" to "Purchase": Once customers add a product to their cart, there is a higher likelihood that they will proceed to checkout and complete the purchase. This is evident from the relatively high retention rates in the "Add to Cart" to "Purchase" stages.
Improving Checkout Process Over Time: There's an increasing retention rate from the "Checkout" stage to "Purchase" in later cohorts (e.g., from 2020-08 to 2020-10). This could indicate improvements in the checkout process, reduced cart abandonment, or effective remarketing campaigns targeting customers who abandoned their carts.
Retention Variability: Some stages, like "Checkout", show a wide variability in retention rates across different months. This could be influenced by factors like promotions, website usability changes, or seasonal shopping trends.
Data Sparsity in Later Cohorts: The most recent cohorts (e.g., 2020-11 and 2020-12) have fewer data points for later cohort indices. This is because these cohorts haven't been around long enough to produce retention data for many subsequent months.
Recommendations:
Optimize the Browsing Experience: There's a significant drop-off from the "Landing" stage to the "View Product" stage. Enhancing product discoverability, offering personalized product recommendations, and improving site search functionality can engage users more effectively and reduce this drop-off.
Streamline the Checkout Process: While the retention rate from "Add to Cart" to "Purchase" was relatively high, there's still room for improvement. Simplify the checkout process, offer multiple payment options, provide transparent shipping costs, and reduce the number of steps to complete a purchase.
Reduce Cart Abandonment: Implement strategies to reduce cart abandonment, such as cart recovery emails, exit-intent pop-ups offering discounts, or reminders for customers who have items left in their carts.
Phew that’s a wrap folks 🍻
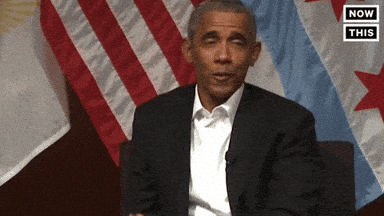
Gif by nowthis on Giphy
Conclusion
However, the journey doesn't end here. Cohort analysis is a versatile tool, with its applications extending far beyond the broad strokes of the customer journey. By diving deeper, ecommerce stores can segment their cohorts in myriad ways, targeting specific niches, behaviors, or preferences. Whether it's focusing on a particular age group, geographic region, or those who interacted with a specific marketing campaign, segmenting cohorts can provide even more nuanced insights.
In our upcoming Part 2, I’ll delve into these advanced applications of cohort analysis. I’ll continue the case study so we can explore how narrowing down on a niche by segment can empower this store to cater to specific customer groups more effectively, optimizing marketing strategies and enhancing customer satisfaction.